Dynamic scenes
![]() |
---|
Frames from sequences used for evaluating performance in dynamic environments from the Bonn and TUM RGB-D datasets |
Most SLAM algorithms have an in-built assumption that the environment will be static. In reality, the vast majority of SLAM use-cases take place in environments with dynamic objects. Dynamic objects are not only a perturbation to be dealt with but often the most important elements of a scene: humans, vehicles, tools, agents able to modify other aspects of the environment. It is thus crucial for a robotic system that they are recognized and modelled appropriately. From the point of view of camera localization, the static world assumption implies that all changes from one frame to another can be accounted for by a single causal factor: camera position. Systems which work under this assumption will therefore include changes produced by dynamic objects when computing the camera pose, and perform erroneously.
Algorithms with RGB-D support are evaluated on the 24 dynamic sequences of the Bonn dataset and the 8 dynamic sequences of the freiburg3 TUM dataset.
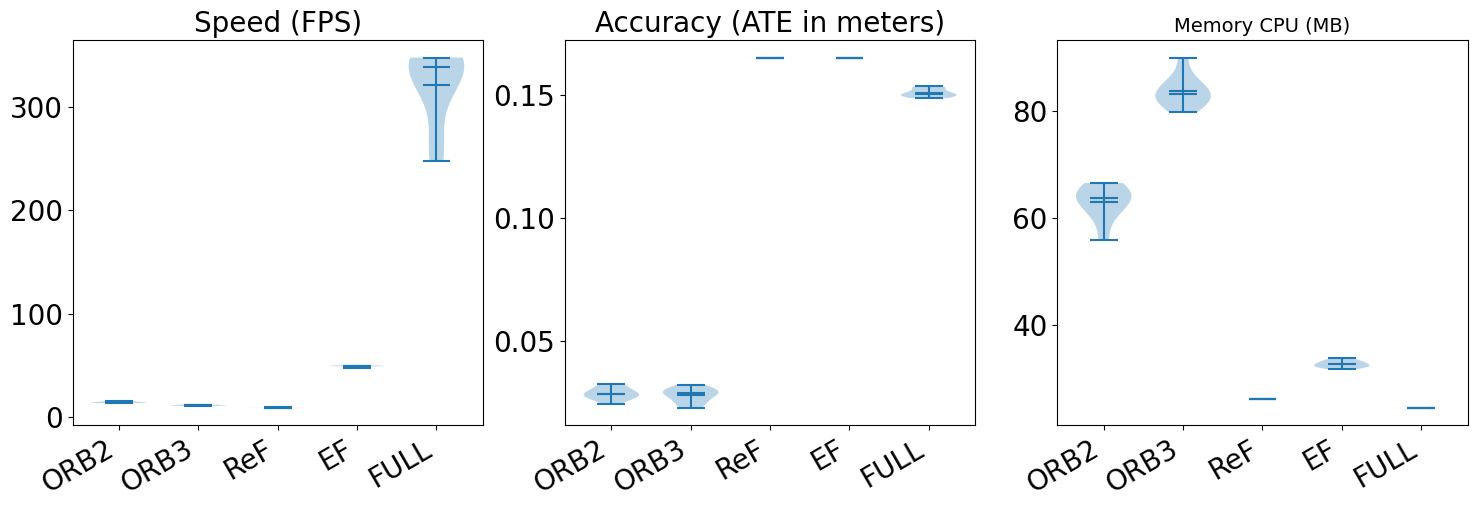
Balloon
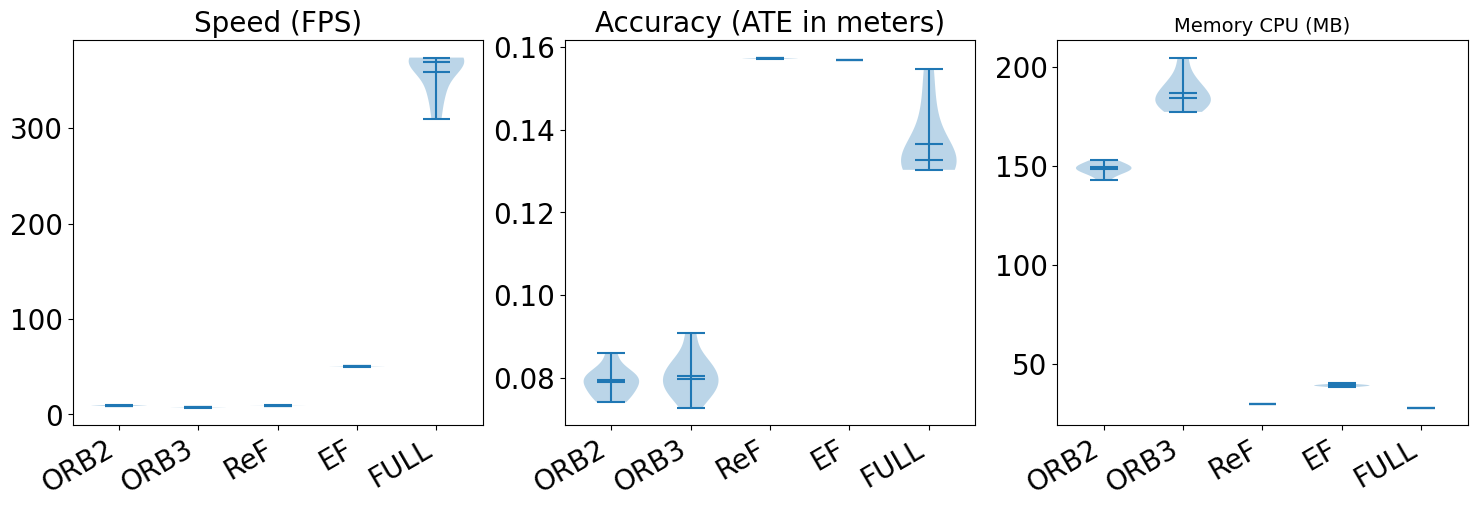
Balloon 2
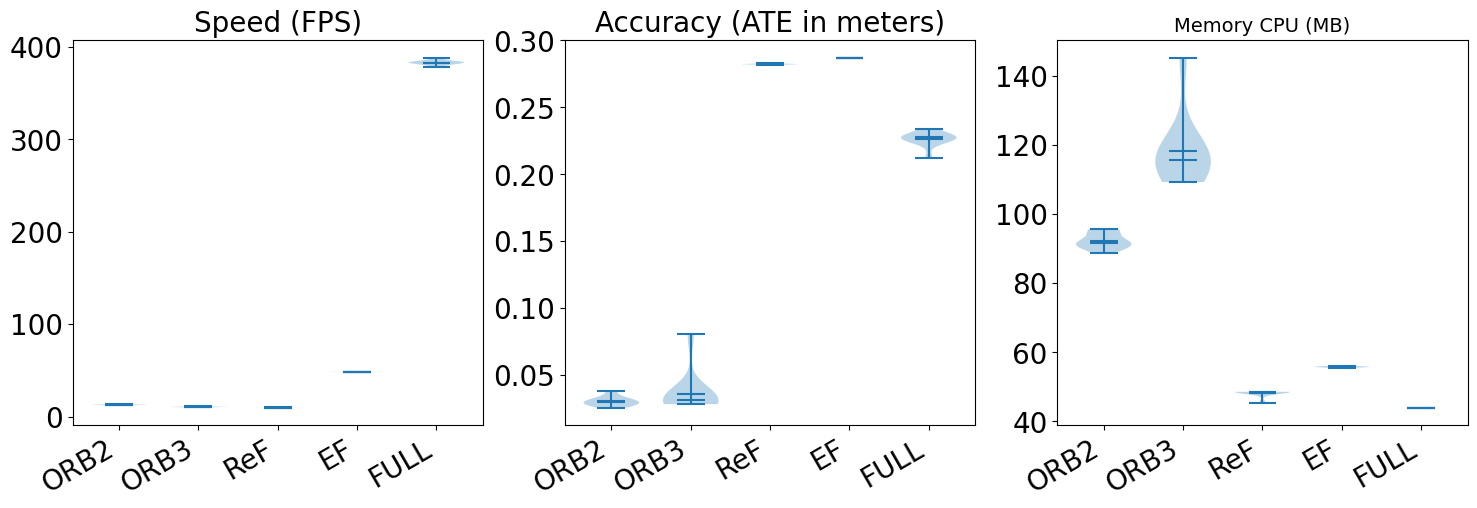
Balloon tracking
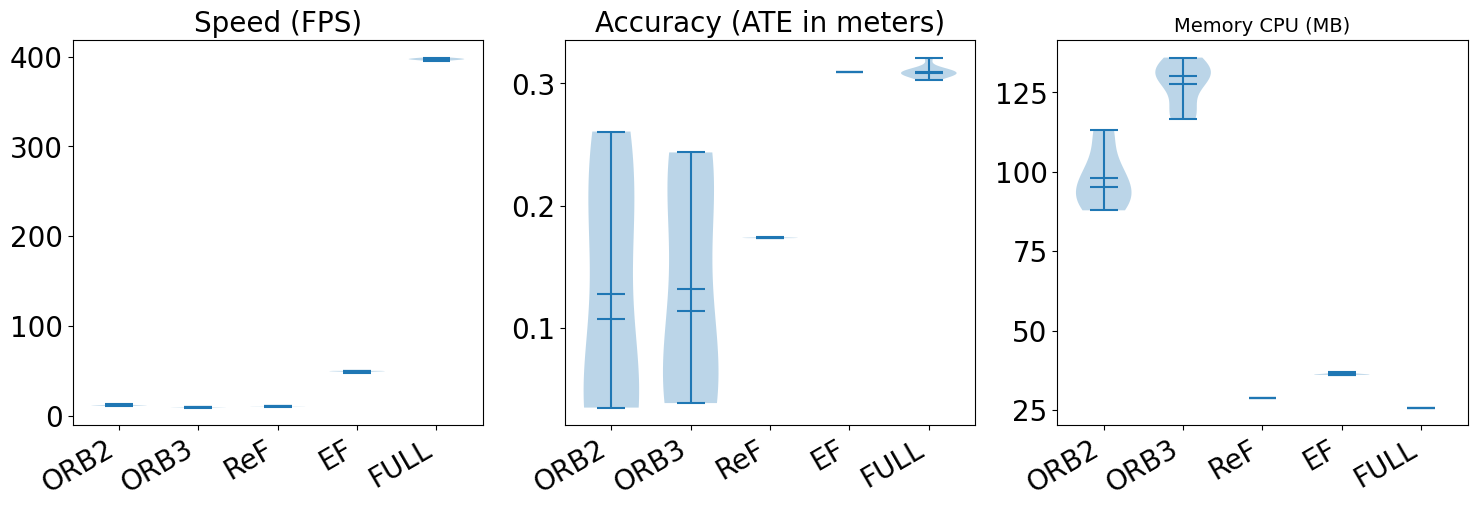
Balloon tracking 2
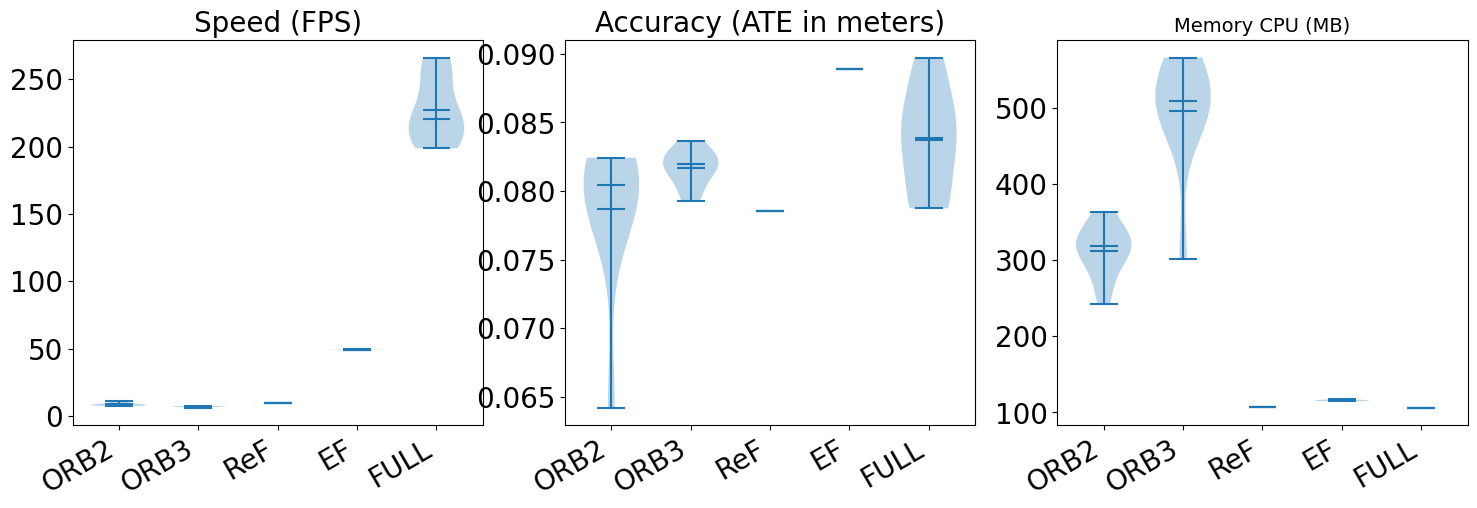
Crowd
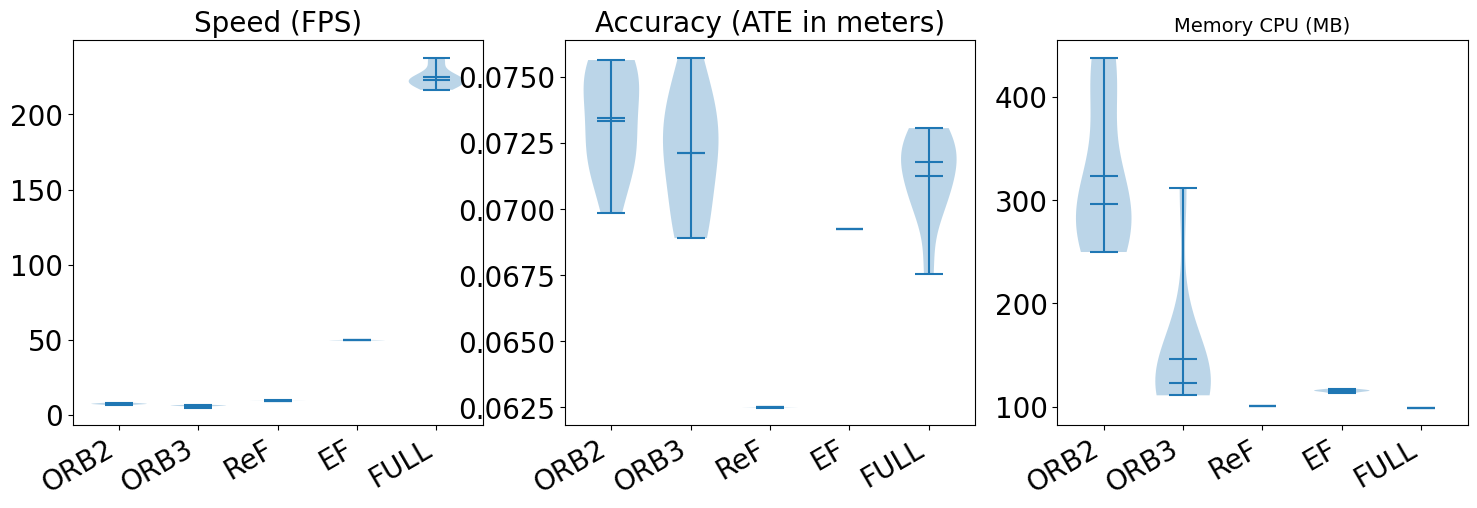
Crowd 2
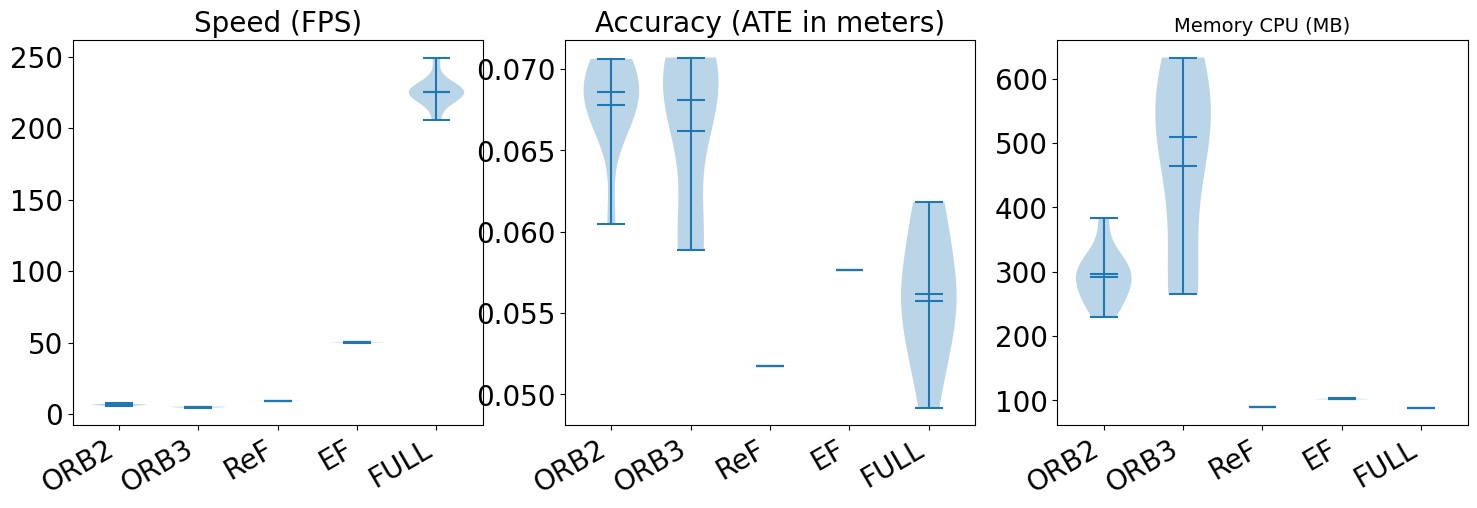
Crowd 3
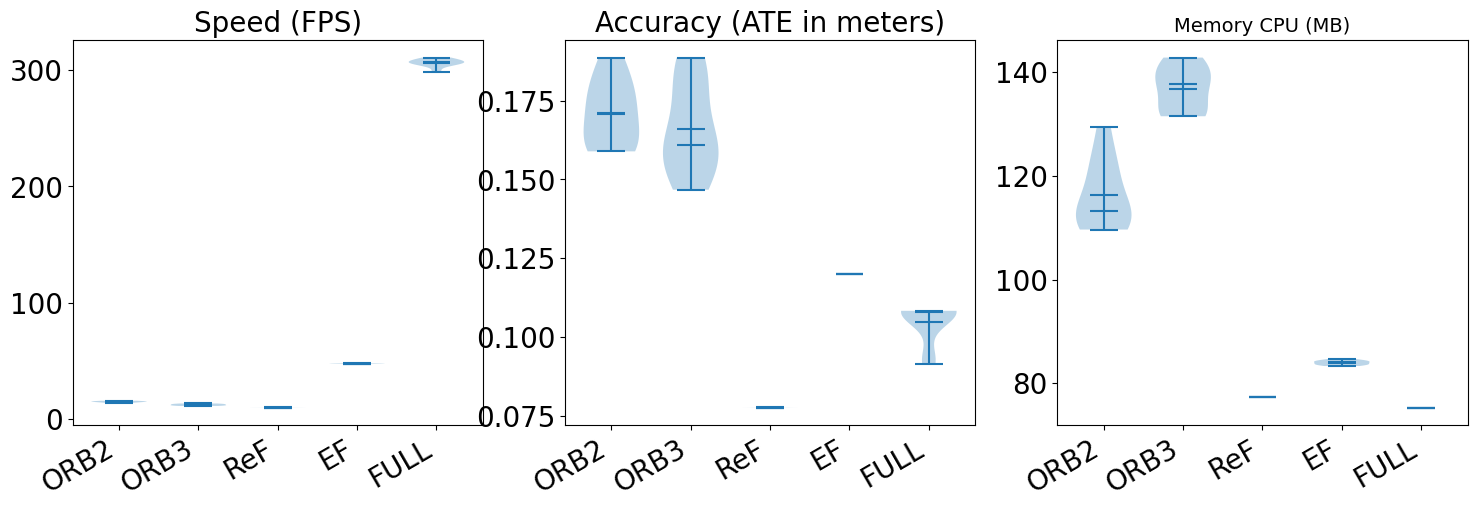
Moving nonobstructing box
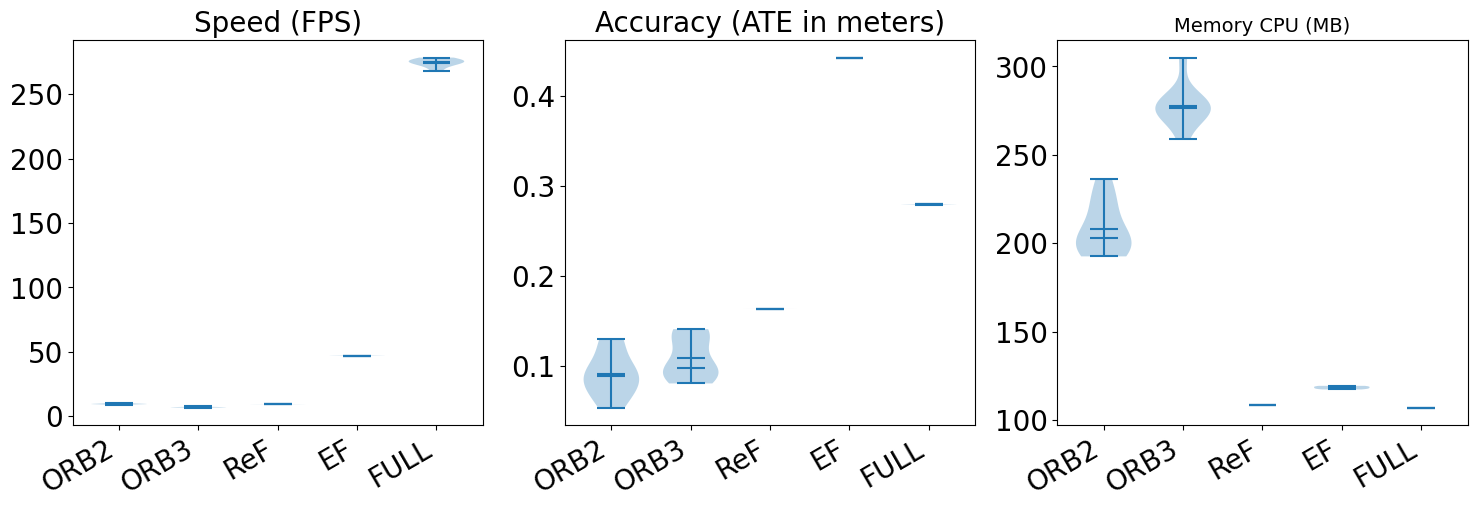
Moving nonobstructing box 2
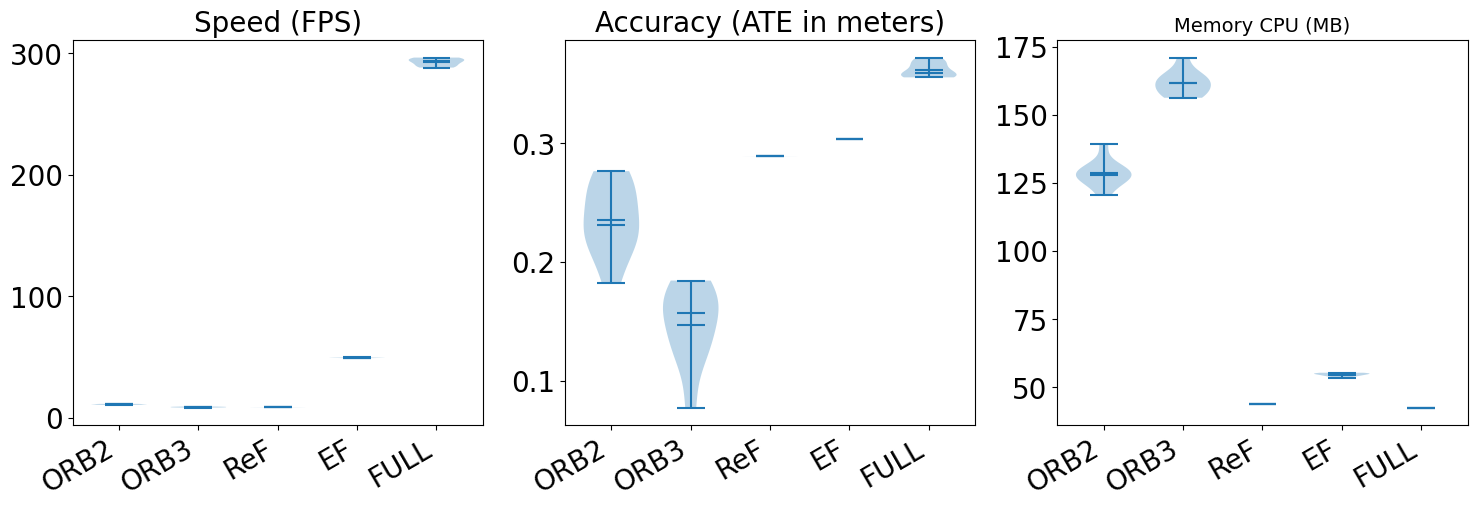
Person tracking
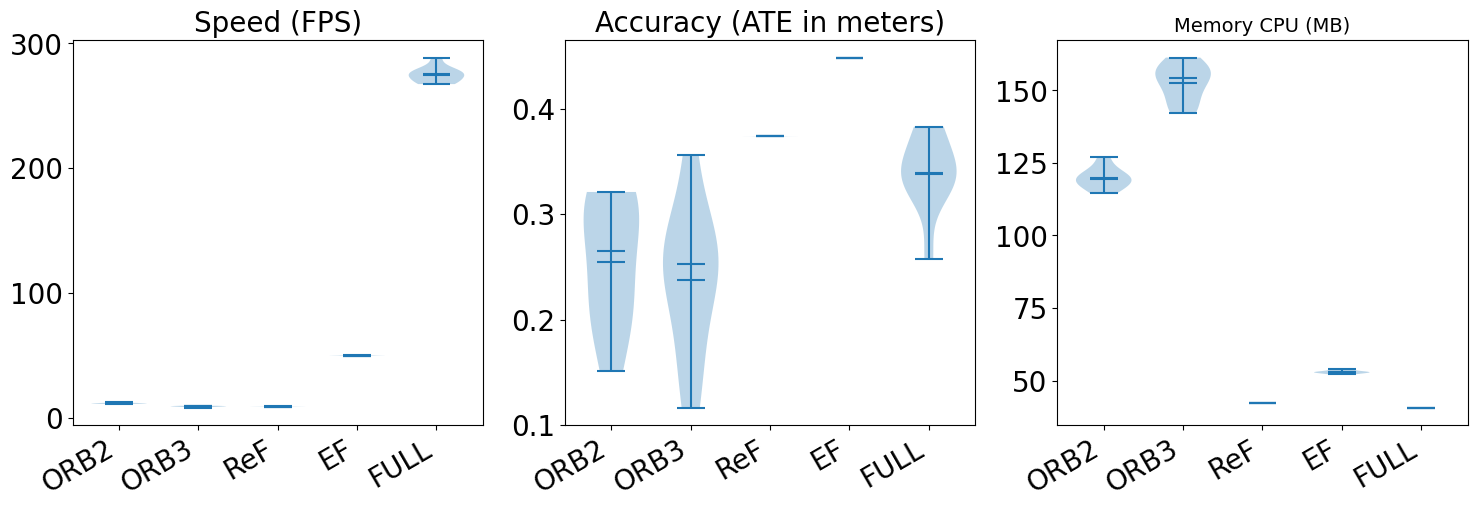
Person tracking 2
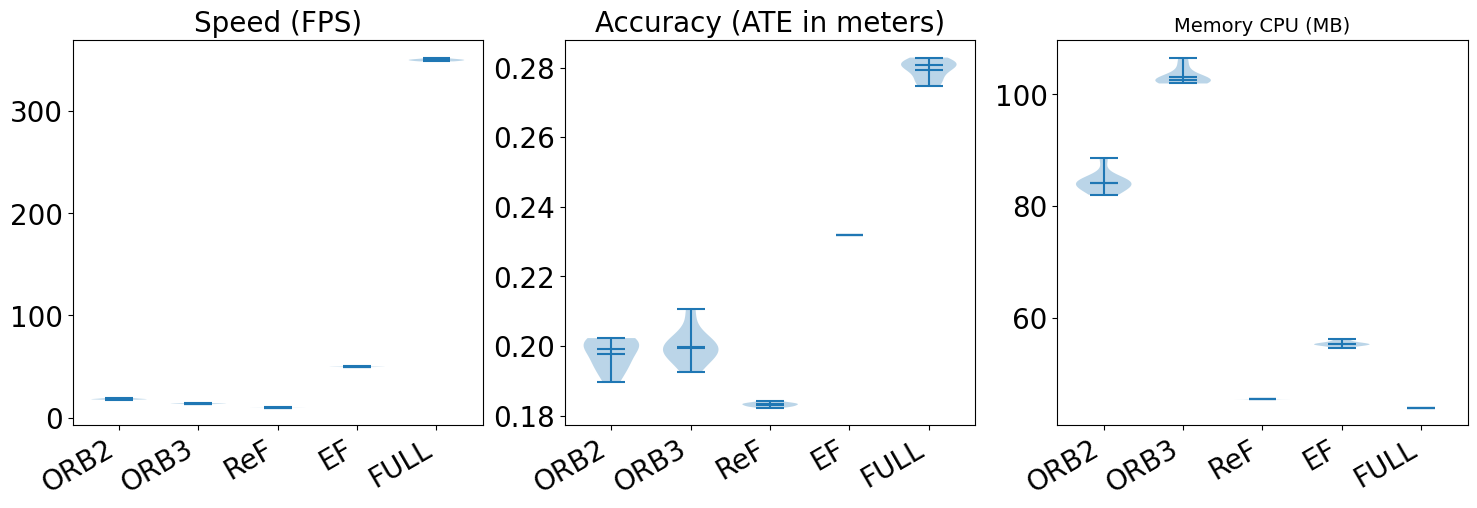
Moving obstructing box
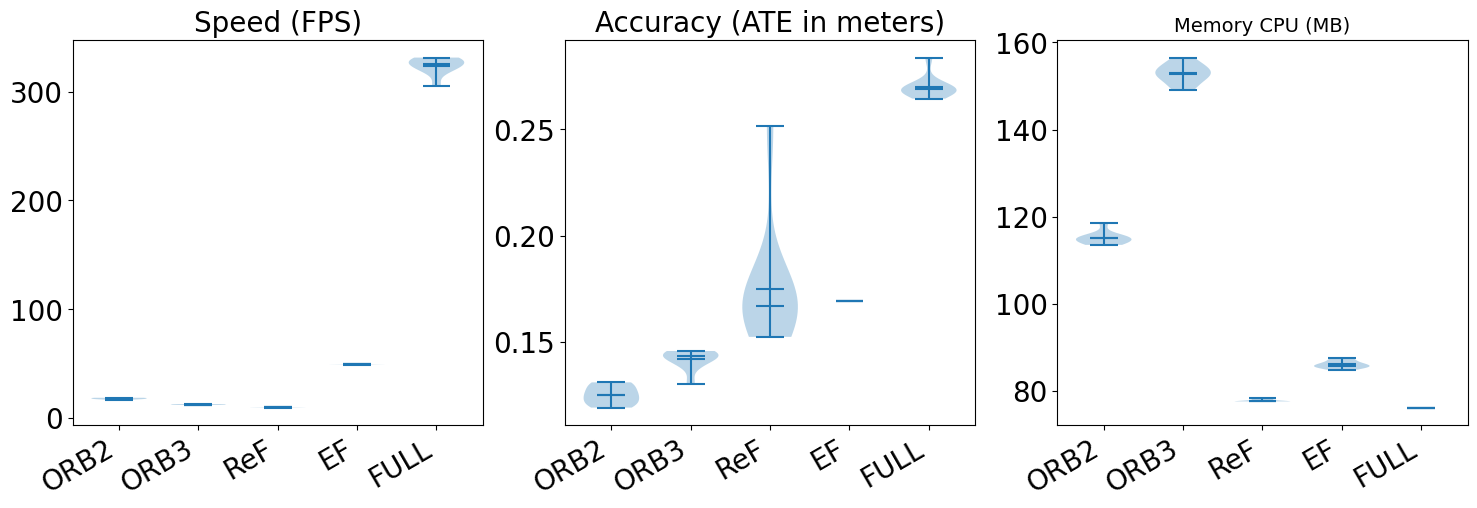
Moving obstructing box 2
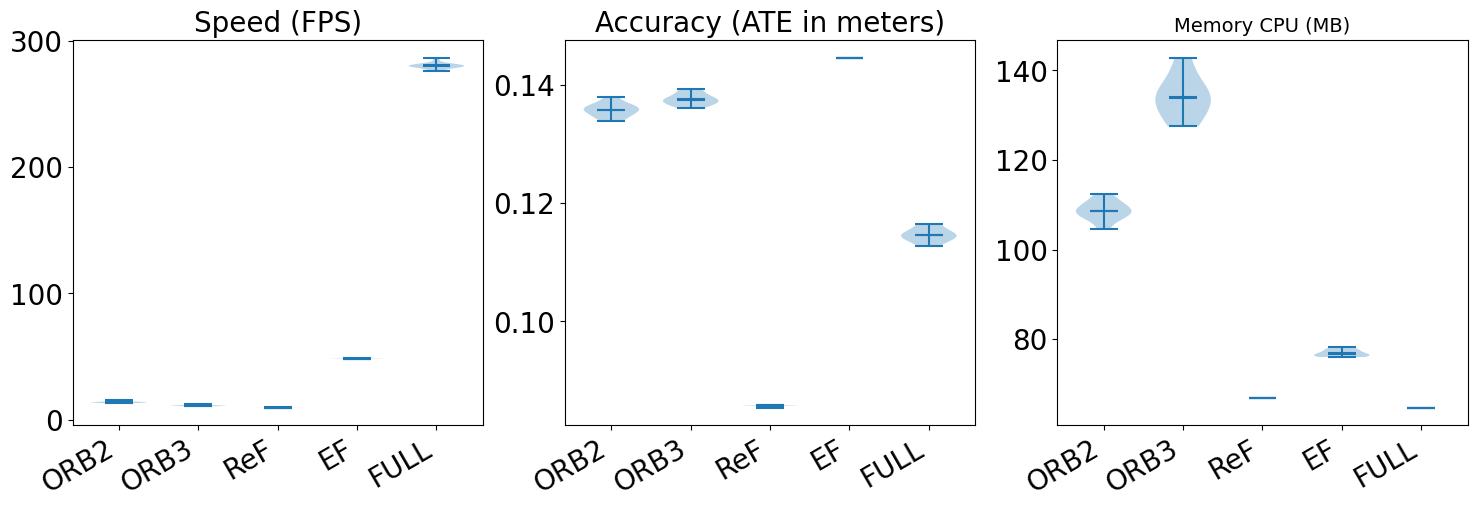
Placing nonobstructing box
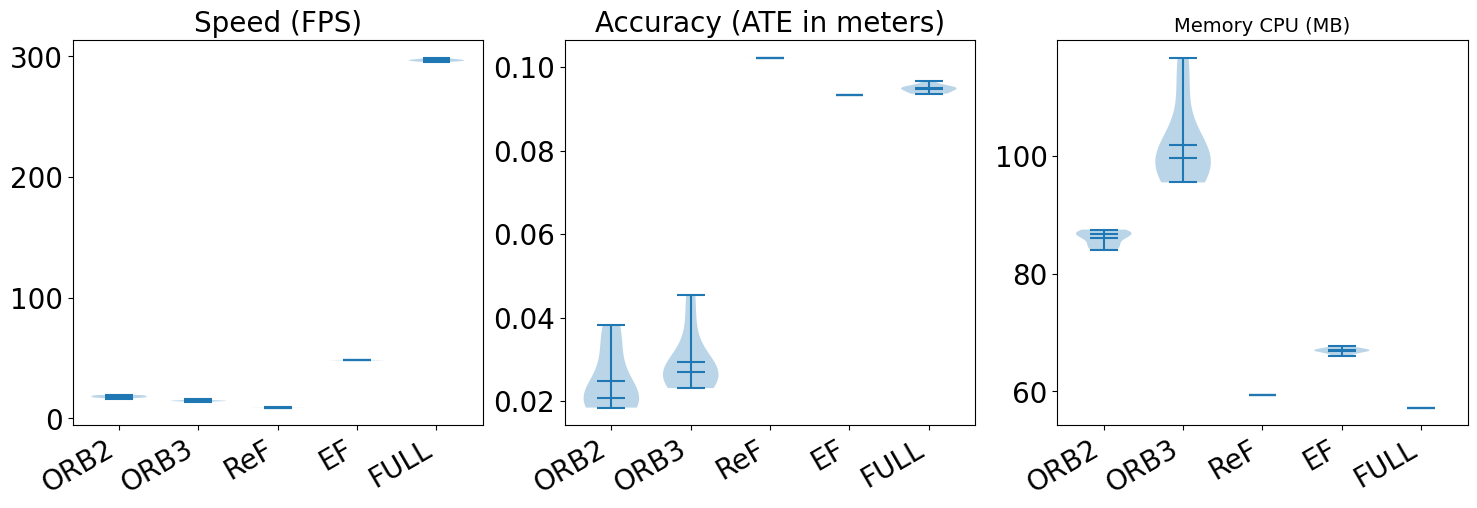
Placing nonobstructing box 2
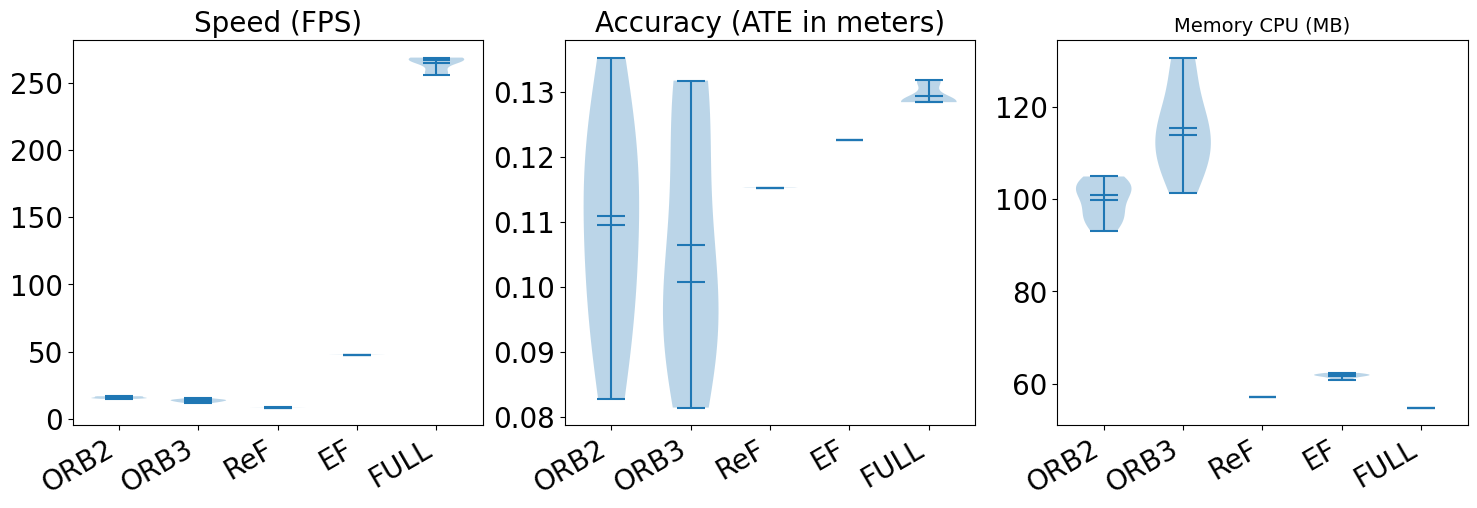
Placing nonobstructing box 3
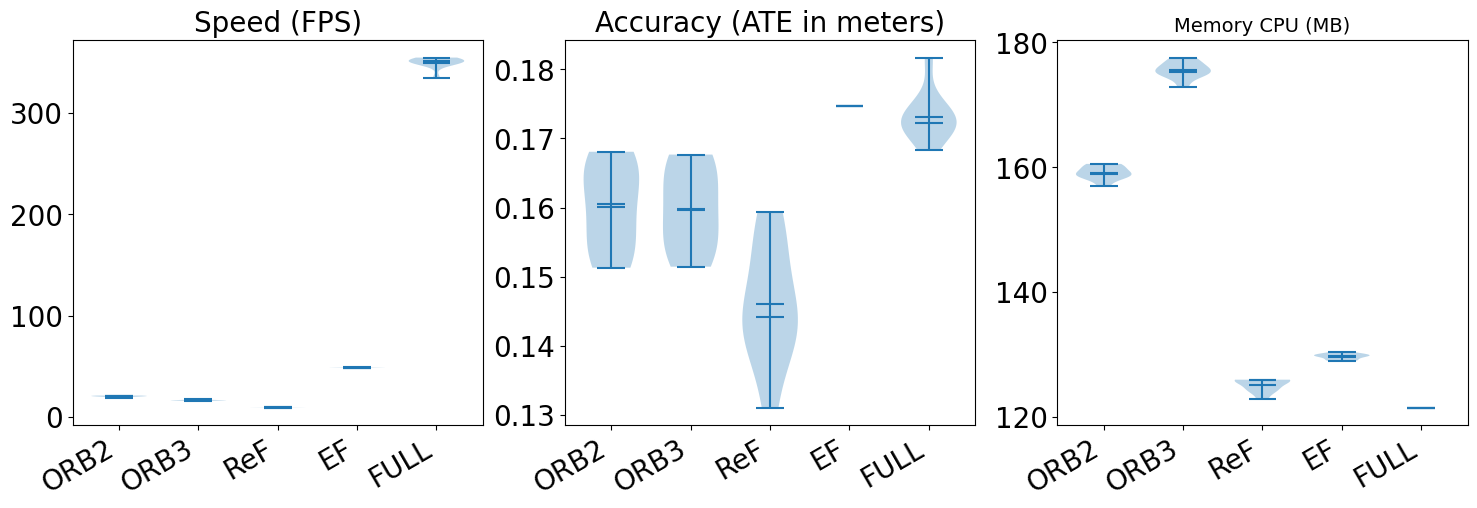
Placing obstructing box
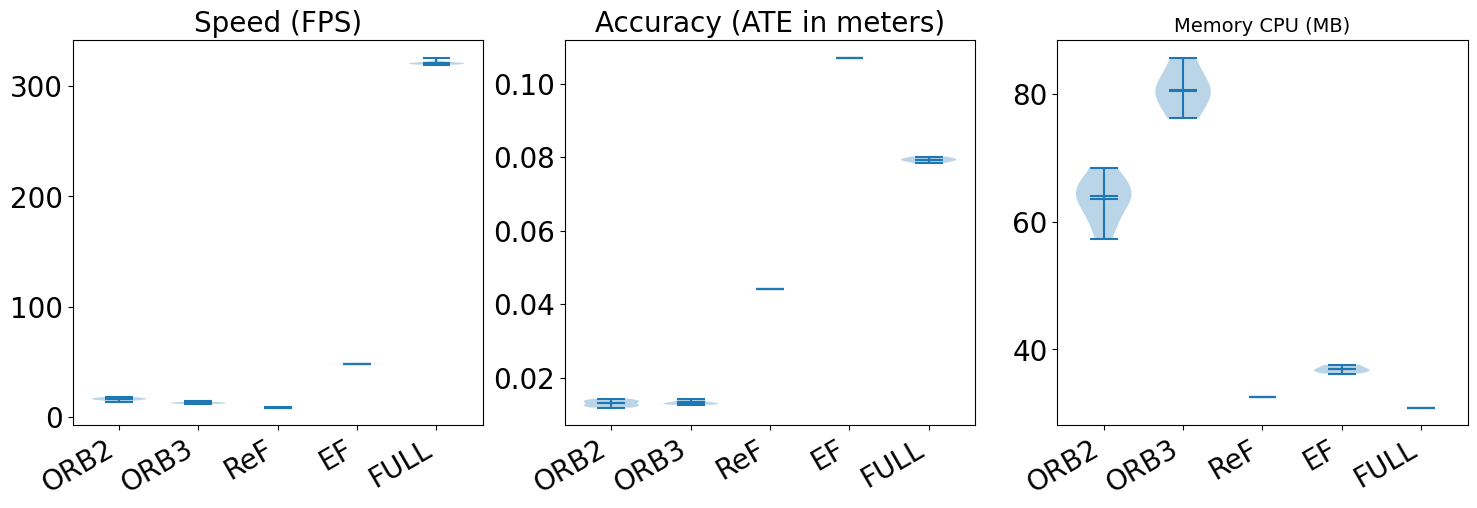
Removing nonobstructing box
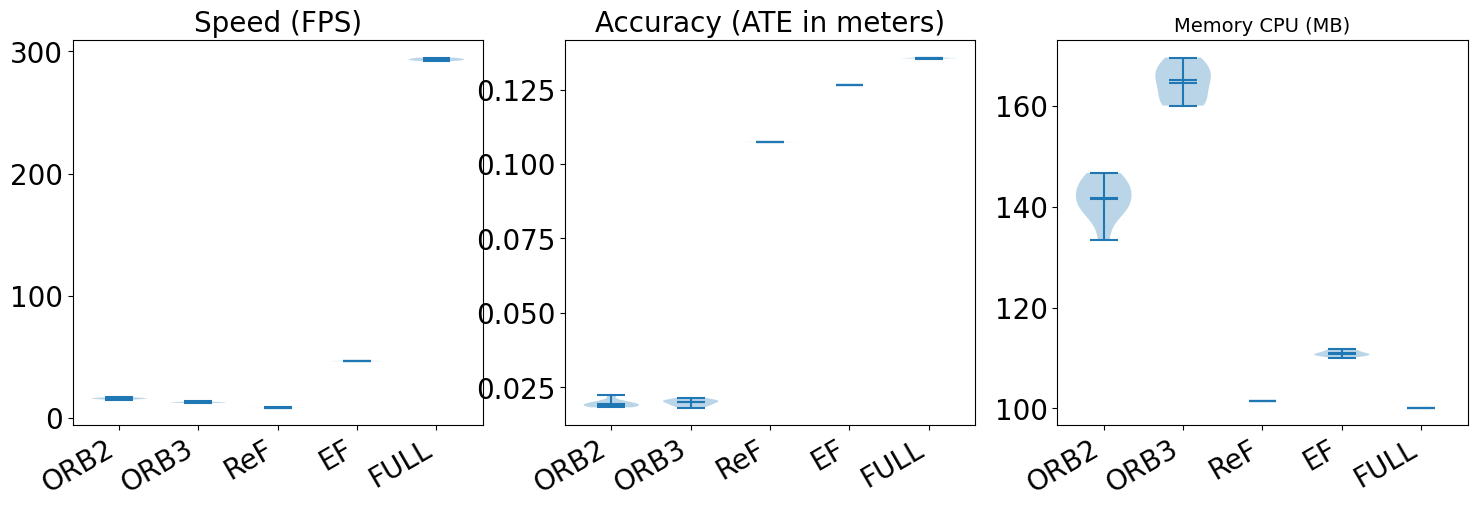
Removing nonobstructing box 2
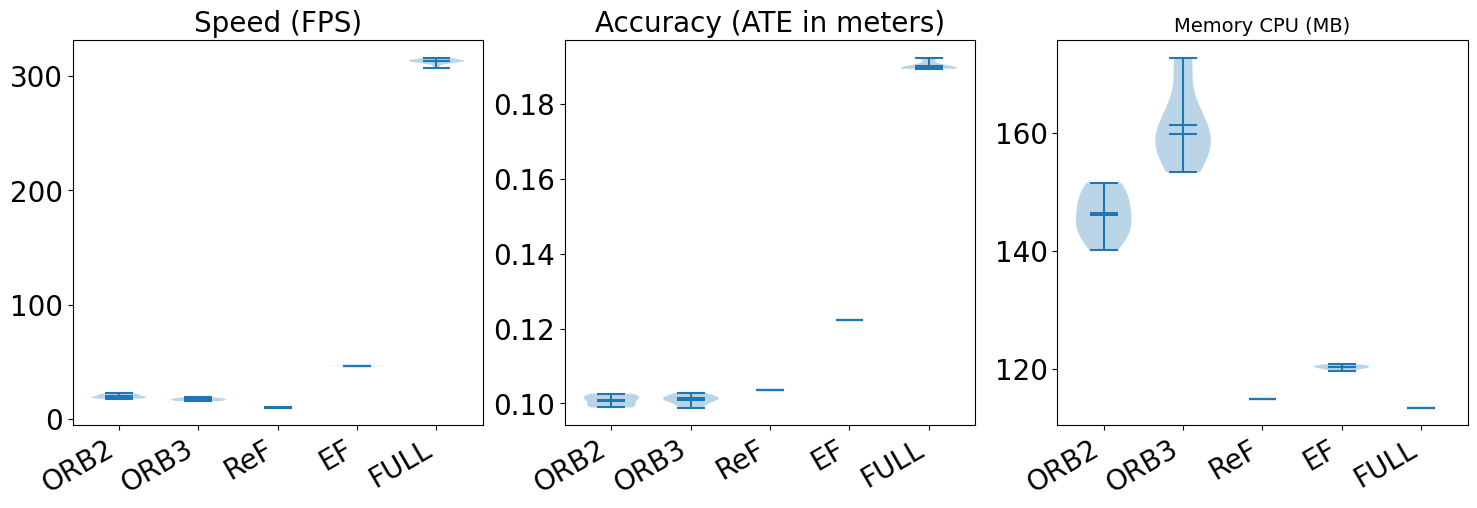
Removing Obstructing Box
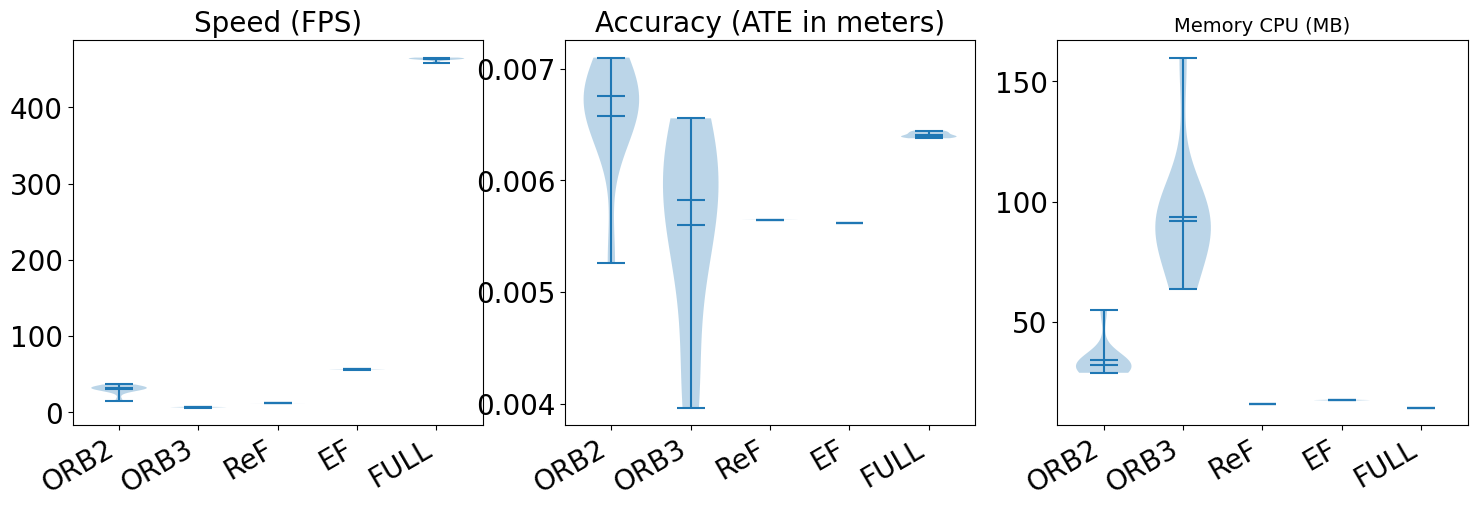
Synchronous
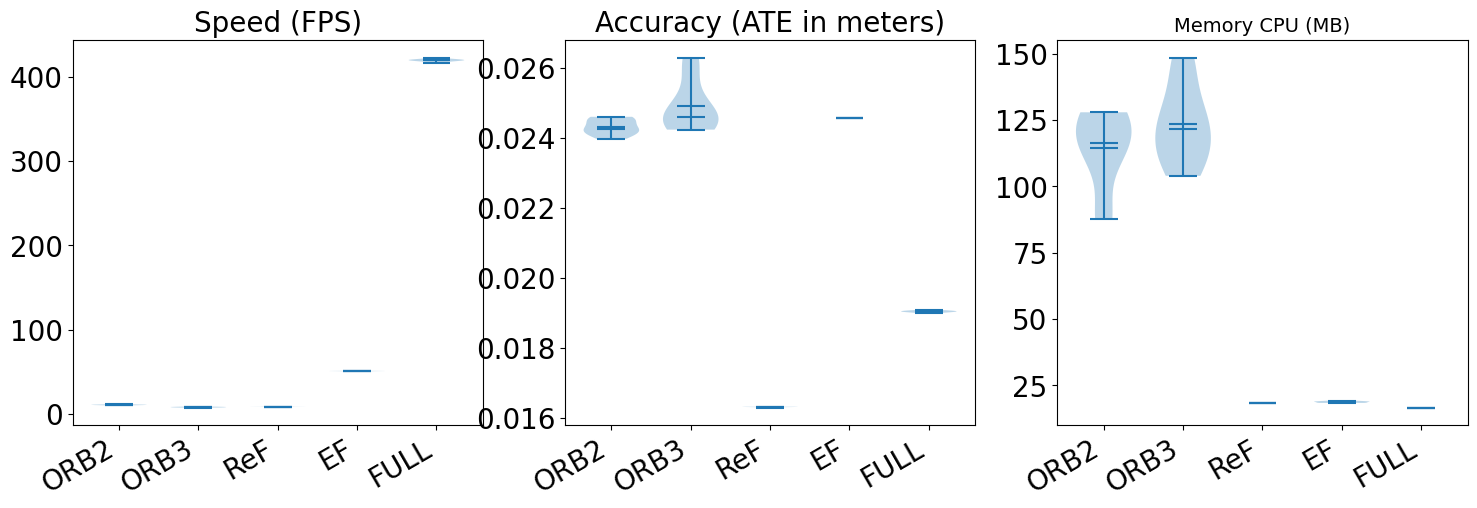
Synchronous2